Policies
"Houston, we have a problem."
-Apollo 13
It would be impossible to efficiently monitor multiple models and model metrics in production by simply observing each model and segment to find potential issues. That's why Superwise provides a flexible monitoring policy builder that lets you express what you want the platform to scan and monitor for on an ongoing basis.
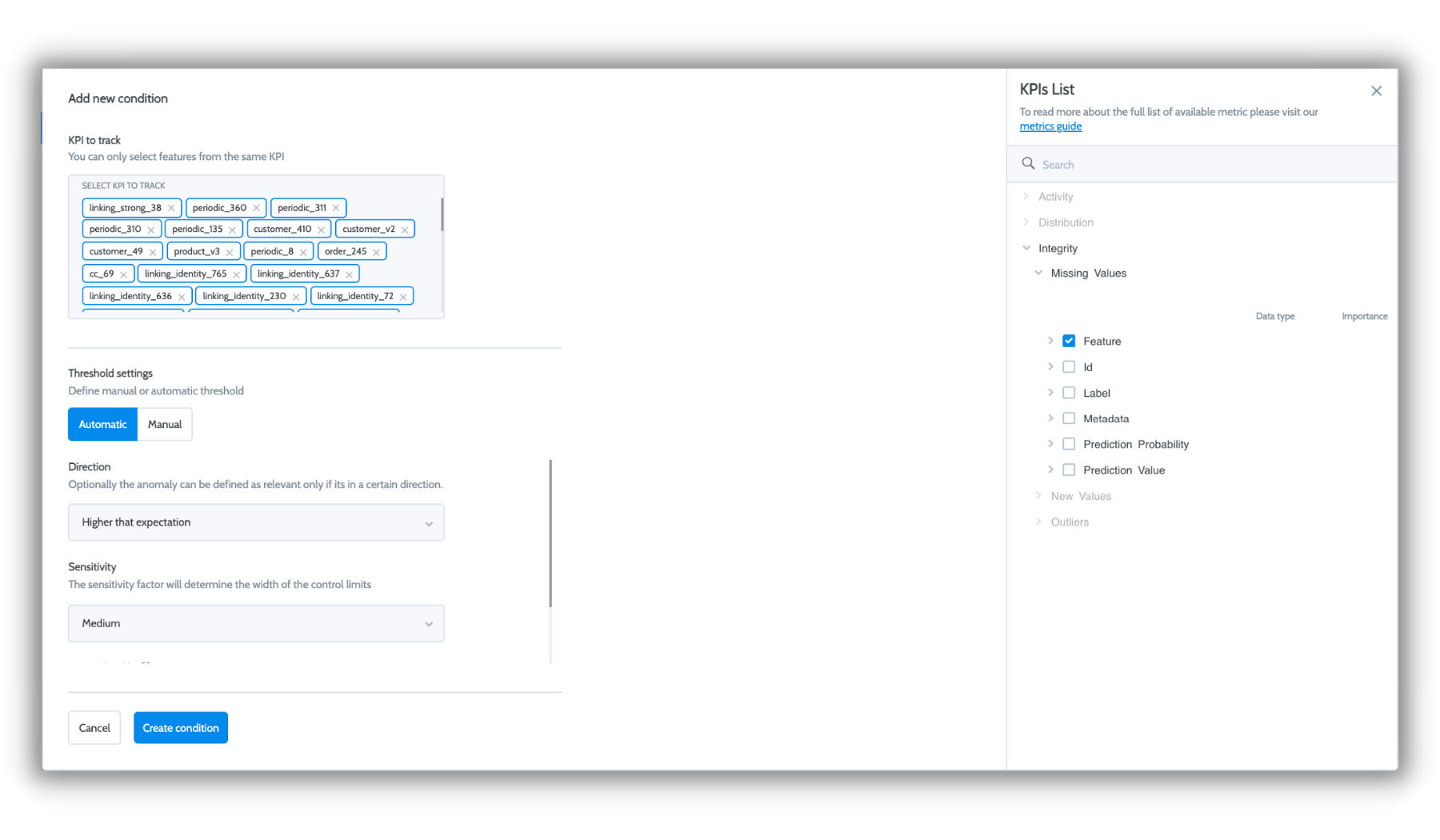
Conditions
A monitoring policy can apply conditions to a specific ML data entity or any combination of entities.
The platform gives users the option to define conditions manually based on thresholds, for example: β% of missing values of all features should be under 5%β. However, no one wants to configure policies metric by metric. It's slow, it's tedious, and it's not scalable. Manual conditions are difficult to define, can have different levels for different segments, and ignore potential seasonality. Superwise's anomaly detection detects abnormal behaviors relative to the normal process behavior in a specific segment. For example: βSearch for anomalies in % of missing values in any of my features.β
Notification channels
Besides its conditions, policies let users define specific team notifications when policies are violated. Notifications can be sent to a particular team or particular channel or any combination of relevant channels and teams. For example, a policy that enforces and checks the level of missing values in the model features may send notifications to the ML engineering teams, while a policy that detects a change in model probably output may inform the DS team and the relevant business operation teams.
Updated over 3 years ago