Data Quality
Data quality
Data quality monitors enable teams to quickly detect when features, predictions, or actual data points don't conform to what is expected.
Superwise offers you a number of Data quality monitoring policy templates:
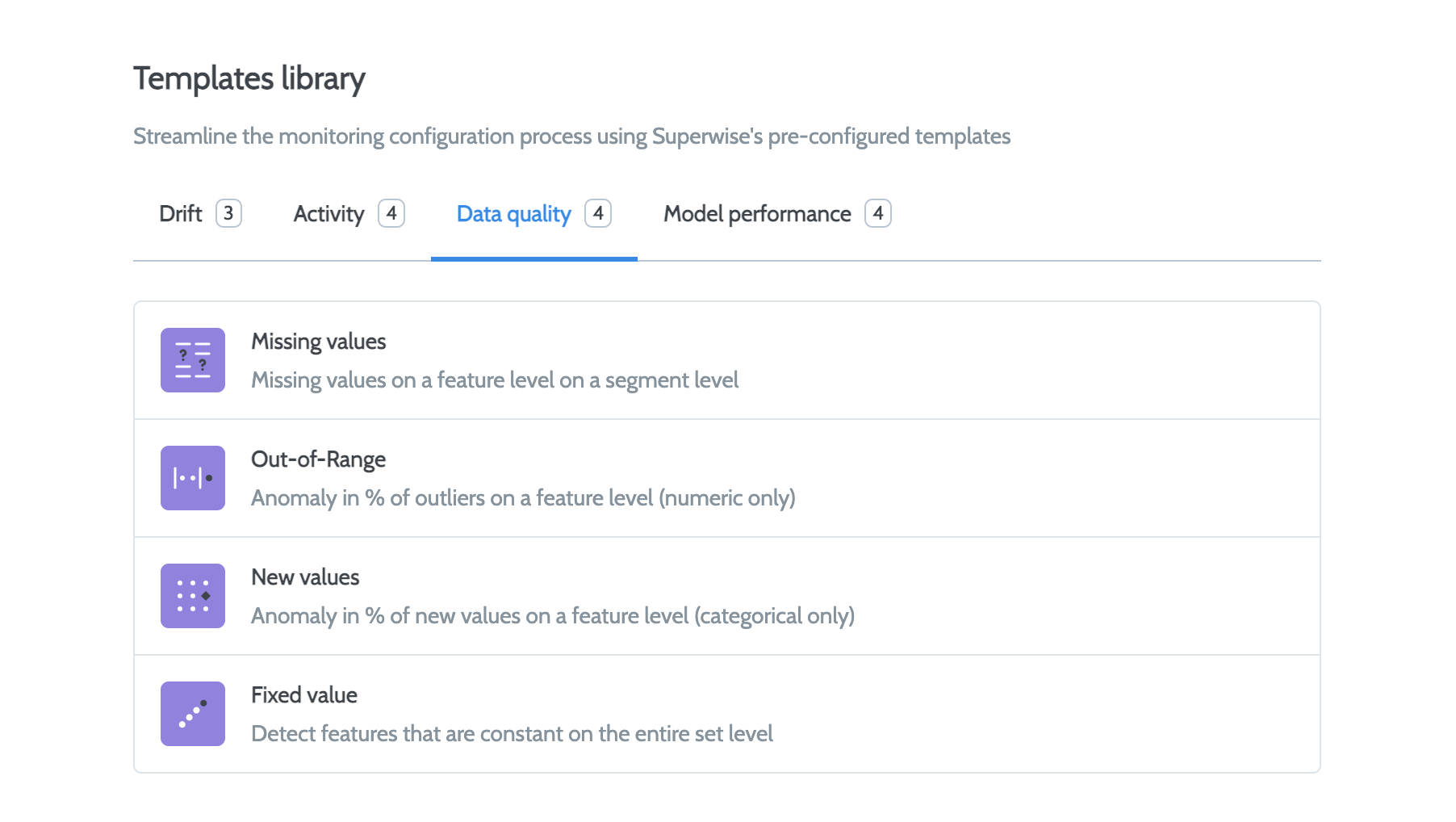
Missing Values
You can monitor for missing values at the feature level for any segment.
Metric | Role | Segments |
---|---|---|
missing values | All Features role | Entire set |
Out-of-Range
You can monitor for anomalies as a percent of outliers at the feature level (numeric only).
Metric | Role | Segments |
---|---|---|
outliers | All Numeric Features | Entire set |
New Values
Superwise can also monitor anomalies as a percent of new values at the feature level (categorical only).
Metric | Role | Segments |
---|---|---|
new values | All Categorical Features | Entire set |
Fixed Value
detect features that are constant on the entire set level
Metric | Role | Segments |
---|---|---|
std / entropy / prop | All Features role | Entire set |
Updated over 3 years ago