Activity metrics
Log activity metrics for your data
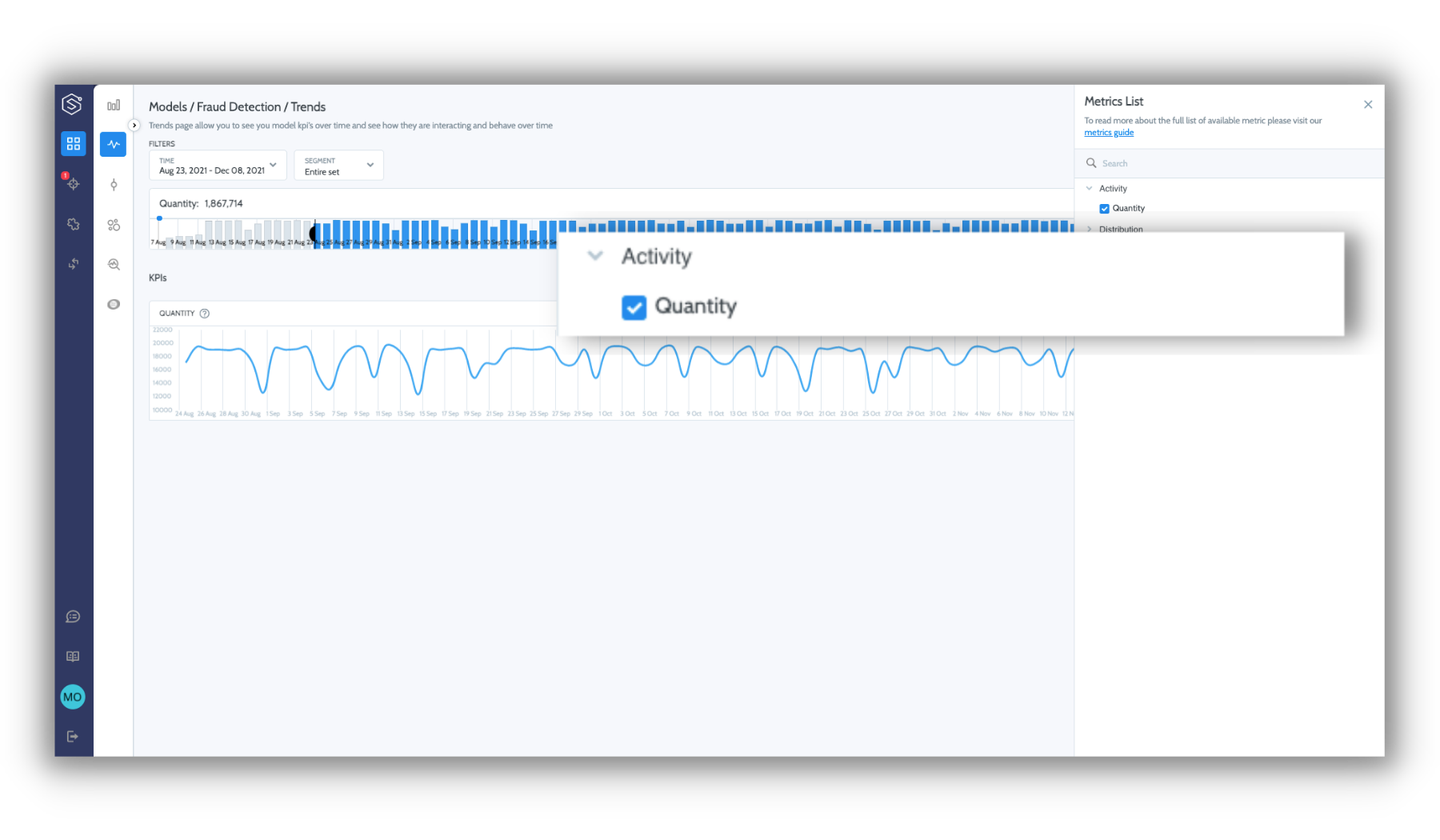
It is vital to measure the activity level of your model and its operational metrics, as variance often correlates with potential model issues and technical bugs.
Superwise currently measures the activity level of your model, meaning the number of predictions and labels that were logged.
Updated over 3 years ago